AMA Interview with Prasad Bhandarkar, Head of Search & Profile Data Engineering, Facebook
As part of the Institute of Product Leadership’s continuous effort to engage senior executives in the industry to share their knowledge and experience with the community, we are kicking off the AMA series (Ask Me Anything) with Prasad Bhandarkar, Head of Search and Profile Data Engineering at Facebook.
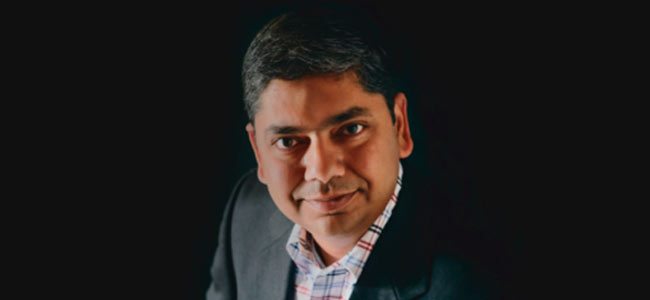
Prasad is Head of Search and Profile Data Engineering at Facebook. Prior to that, Prasad was instrumental in developing and realizing next generation data strategy at Adobe as the company transformed its business model to cloud subscription. Prasad is a certified executive coach and helps fellow engineers, product leaders and data professionals realize their potential.
Who better than Prasad to get our burning questions about data science answered. So, that’s what we did and Prasad was kind enough to take some time to share his thoughts and wisdom. If you have more questions, feel free to ask them in the comments to this post.
Q: IPL: What is the role of Data Science in enabling a product’s growth?
Having data driven insights about a product is akin to having someone shine a lantern on the road in the dark night. Absent data insights and data science, product management would be blind to user behavior and interaction with products. For example, one would not know which features are most useful to customers and which are not.
Product management is about making bets about the product and by definition there is an art to it. Data informs and fine tunes this art to make the product resonate with the users.
In old days, some of this used to be done through user focus groups but that of course, had limitations of velocity and depth. With modern data science, one can slice, segment and pinpoint insights at rapid speed and help product iterate at very short cycles. In other words, data science closes the feedback loop and unlocks insights that shape the direction of products.
Finally, data science can enable hyper personalization of the product, making it relevant and interesting. There are several examples of this in some of the favorite products we already use – recommendation engines in Amazon, Netflix and YouTube for example. In all these cases, signals from past customer behavior and stated preferences are used in intelligent ways to change product behavior to be most useful to that customer.
Q: How do data scientists collaborate with other team members? (Engineering, product management, marketing, etc.?
Data scientists are co-equal partners with product management, engineering, data engineering and design teams. They are experts in keeping the process honest. They generate insights into what is working, what is not working and what could work.
The term co-equal here is used in the same spirit as it is used in the US Constitution. Data Scientist are complementary – and not subservient – to product management. They collaborate with product management on product strategy and insights, but also have a role to keep product management honest and shine bright light on any blind spots. Data scientists may – for example – prove that certain aspect of product strategy has hit a dead end in spite of best attempts, and it may be time to kill it. In lot of ways, this offers check and balance in the system about objectiveness of product success.
For example, let’s say that product management of a certain video streaming site decides to add in video advertisements in the middle of content. Data scientists can help them to decide optimum placement of ad, optimum content to ad duration ratio, or Data scientists may also find that while such ads may drive up revenue they may drive down engagement and retention and therefore, may not be a good idea after all as a matter of overall product strategy. Or they may determine that these type of ads are appropriate only for certain type of content and not others.
These insights form a crucial basis for driving the direction of the product.
Q: What are your favorite tools and techniques for data analysis?
Like many other areas, data science is not just about statistical techniques. It is about forming well-defined hypotheses. It is about doggedly asking clarifying questions to get clarity on what it exactly one is trying to achieve from an analysis. If this is done right, in most cases half the problem is solved right there as it would prevent unnecessary churn. It would also provide clarity on which tools and techniques may be appropriate to prove or disprove the hypothesis.
Interestingly, data scientists more than often have to deal with imperfect data. This may be due to several reasons – recent instrumentation of data and therefore inadequate history, missed events due to technical reasons (it is generally followed practice to trade off reliability of events collection in favor of customer experience if such a tradeoff needs to be made), sample size tradeoffs when it comes to humongous data volumes etc. Statistical techniques allow us to address and help mitigate such imperfections.
Driving decisions and insights based on imperfect data and using appropriate statistical techniques for that is an important tradecraft for a good data scientist.
Q: What are your top tips for getting hired as a data scientist?
As I described above, probably the most important part of being a good data scientist is to have ability to develop solid hypotheses. This ability requires product intuition and curiosity. In lot of ways, a data scientist should be able to think like a product manager and should be familiar with key product management concepts like segmentation, targeting, positioning etc.
Of course, having proficiency in statistical methods is table stakes for these roles. Having proficiency on common data platforms (Hadoop/Hive, Presto, Spark etc) as well as common languages (SQL, Python, R etc) is also table stakes.
It is important to put all this together and not just learn theoretically. An aspiring data scientist may well be advised to practice the end to end tradecraft using publicly available datasets. In a typical data science interview, a good interviewer will likely ask and expect a solution to an end to end problem.
Therefore, in addition to preparing on statistical techniques, think of an end-to-end problem – such as business models of well-known product companies – Netflix, Twitter, AirBnB, Lyft etc. You can then think about how you will measure success of these business models if you were running these businesses. What metrics will you use? What metrics will you avoid using? How do you make sure that there are checks and balances between metric ecosystems to drive behaviors in line with product strategies? What kinds of new features would you like to see? How can you setup A/B tests to validate contribution of these new features to overall strategy? What will be your hypotheses if you see a sudden uptick or downtick in one or combination of metrics and what techniques and methods will you use to prove or disprove those hypotheses?
Q: How should a company prepare to build out a data team?
The first big difference is what is most important: relationships vs contracts. East vs West, North vs South as a very general approximation. Hofstede and Trompenaars have some useful academic models for understanding cultural differences. However, the key issue is just be aware that the person across the table will be thinking differently and you will have some blind spots, so be ready to be flexible.
Do some research about what is important in their culture and try and watch some famous movies from that culture e.g. I always recommend Lagaan as important to understanding part of Indian culture. Then be friendly, ask lots of questions and be interested in their culture. People around the world are almost always trying to be nice to their guests and appreciate your interest. Above all don’t be patronising – your country’s way of doing things is not the best, it is only one way of solving the problem…it may be relevant, it may not.
Remember that culture gets wrapped around the negotiation process. People are talking to you because you have something they want, and vice versa, so be aware of the well-known cultural issues. Be ready and prepared to negotiate, once they have done the cultural bits and decided if you have good will and they can trust you to behave in a manner that makes logical sense to their cultural lens.
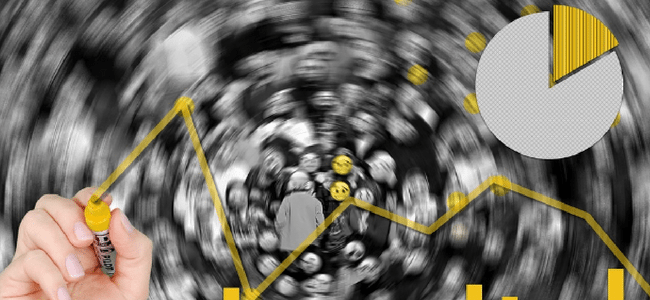
Q: What tips can you share to effectively present data insights to management?
It is easy to get lost in data and dashboards. It is important to remember that ultimately, product or business insights is a story. It has to have a coherent chain of thoughts, and analysis should be supporting the chain of thoughts. In a lot of ways, this is identical to planning, designing and delivering a business presentation. You should ask the question – what are you trying to say – first. That story will be informed by your understanding of what data is saying. However, delivering that story does not mean you deliver the data dump. Instead, pare down metrics to what you think make the point. Use visualization techniques that declutter unnecessary parts and make clear points. Have the side stories in your back up slides, in case they come up.
Q: Top 3 blogs you personally follow.
These three blogs provide wider perspective on data strategy and how business leaders should think about it.
McKinsey on Business – this blog has all sorts of interesting topics, but the ones around AI and Bigdata are more relavant for the topic we are discussing
Andrew Chen’s blog – some great insights into business models and how to think about them
MIT Technology Review – MIT’s blog on technology and its impact on world If you are more technically inclined, you may want to read these
Data Science Central – All sorts of interesting statistical analysis topics
Andrej Karpathy’s blog – Andrej Karpathy is a pioneering Stanford researcher on AI and currently Director of Data Science at Tesla. His blog is uncannily novice friendly.
Finally, if you want to have an overview of neural networks and have fun in the process, try this https://selfdrivingcars.mit.edu
Q: Imagine a person who knows nothing about data science. Where does she start?
Few scenarios/situations
1. If she is interested in just overview and how to think about it at a high level, following blogs like McKinsey, HBR etc should help.
2. If she wants to get deeper into data science, she should first have a good understanding of college statistics (sampling, regression etc). She should learn programming languages like SQL and Python. Some good courses to take after that are – Coursera course by Andrew Ng, MIT’s Big Data and Social Analytics Course etc.